
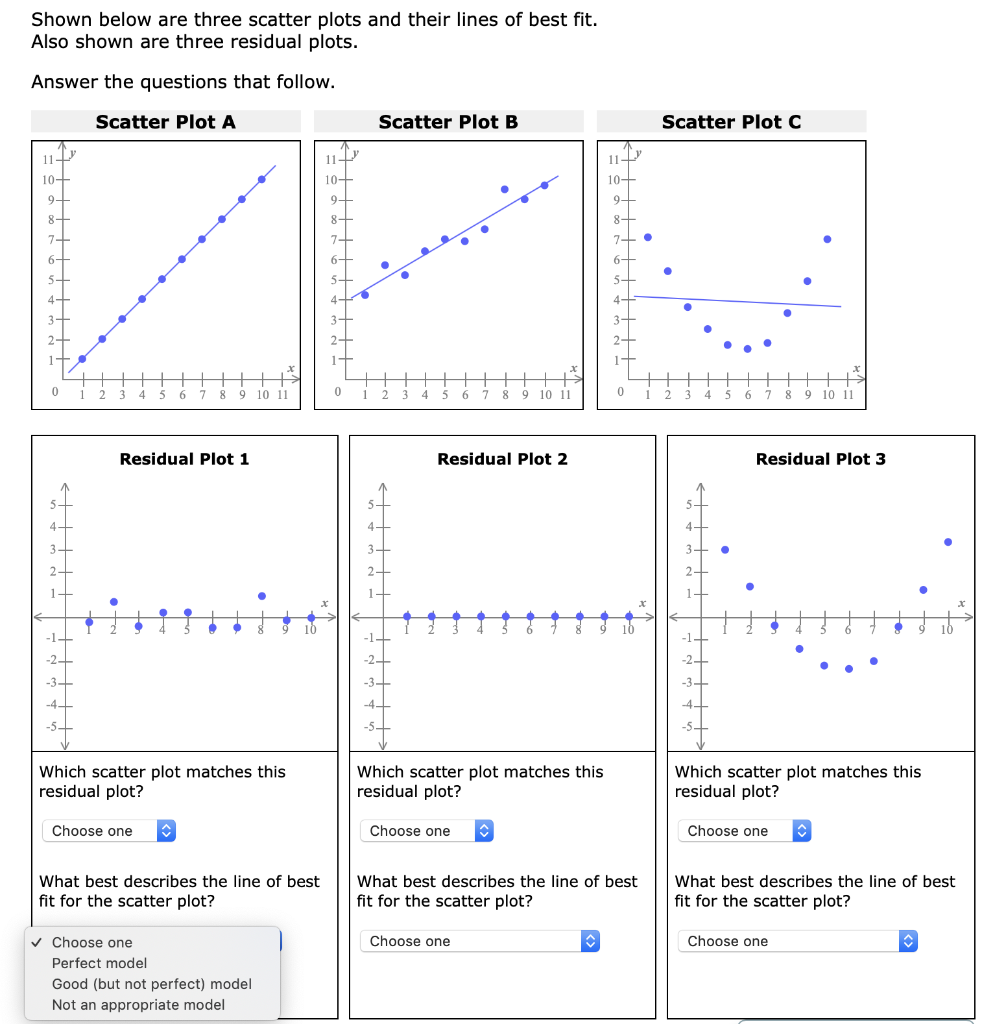
Now look at how and where these five data points appear in the residuals versus fits plot. Also, note the pattern in which the five data points deviate from the estimated regression line. Note that the predicted response (fitted value) of these men (whose alcohol consumption is around 40) is about 14. In case you're having trouble with doing that, look at the five data points in the original scatter plot that appear in red. You should be able to look back at the scatter plot of the data and see how the data points there correspond to the data points in the residual versus fits plot here. Note that, as defined, the residuals appear on the y axis and the fitted values appear on the x axis. Here's what the corresponding residuals versus fits plot looks like for the data set's simple linear regression model with arm strength as the response and level of alcohol consumption as the predictor: And, it illustrates that the variation around the estimated regression line is constant suggesting that the assumption of equal error variances is reasonable. It also suggests that there are no unusual data points in the data set. The plot suggests that there is a decreasing linear relationship between alcohol and arm strength. A fitted line plot of the resulting data, ( alcoholarm.txt), looks like: They also measured the strength ( y) of the deltoid muscle in each person's nondominant arm. The researchers measured the total lifetime consumption of alcohol ( x) on a random sample of n = 50 alcoholic men. Some researchers (Urbano-Marquez, et al., 1989) were interested in determining whether or not alcohol consumption was linearly related to muscle strength. Let's look at an example to see what a "well-behaved" residual plot looks like.

The plot is used to detect non-linearity, unequal error variances, and outliers. It is a scatter plot of residuals on the y axis and fitted values (estimated responses) on the x axis. When conducting a residual analysis, a " residuals versus fits plot" is the most frequently created plot.
